Dataset
We introduce a large-scale photorealistic dataset (ShapeNet6D) to empower the network's generalizability to novel objects. Rendered RGBD images are labeled with instance semantic segmentation and 6D pose parameters.
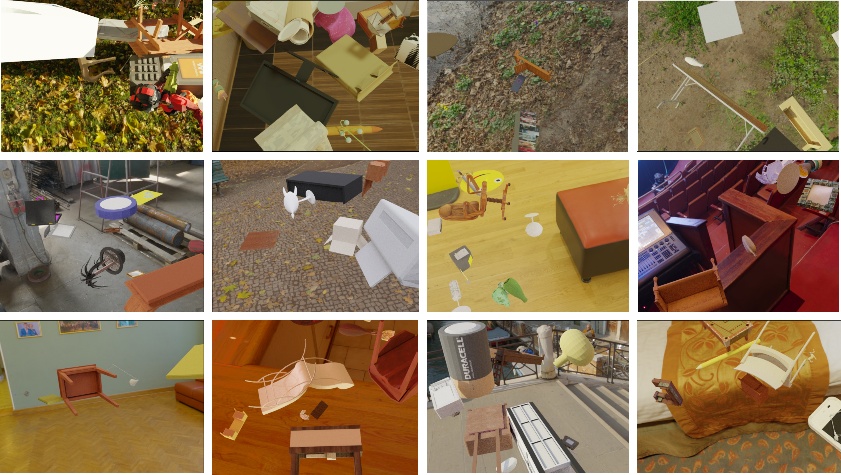
6D object pose estimation networks are limited in their capability to scale to large numbers of object instances due to the close-set assumption and their reliance on high-fidelity object CAD models. In this work, we study a new open set problem; the few-shot 6D object poses estimation: estimating the 6D pose of an unknown object by a few support views without extra training. To tackle the problem, we point out the importance of fully exploring the appearance and geometric relationship between the given support views and query scene patches and propose a dense prototypes matching framework by extracting and matching dense RGBD prototypes with transformers. Moreover, we show that the priors from diverse appearances and shapes are crucial to the generalization capability under the problem setting and thus propose a large-scale RGBD photorealistic dataset (ShapeNet6D) for network pre-training. A simple and effective online texture blending approach is also introduced to eliminate the domain gap from the synthesis dataset, which enriches appearance diversity at a low cost. Finally, we discuss possible solutions to this problem and establish benchmarks on popular datasets to facilitate future research.
We introduce a large-scale photorealistic dataset (ShapeNet6D) to empower the network's generalizability to novel objects. Rendered RGBD images are labeled with instance semantic segmentation and 6D pose parameters.
A Siamese full flow bidirectional fusion network extracts rich appearance and geometric features from the support view and the query scene patch, respectively. They are then fed into self- and cross-attention modules to obtain dense support prototypes and query features to explore correspondence for pose estimation.
Our framework can genralize to novel objects unseeen during training.
We establish benchmarks for the problem on two popular datasets to facilitate future research.
@article{he2022fs6d,
author = {Yisheng, He and Yao, Wang and Haoqiang, Fan and Qifeng, Chen and Jian, Sun},
title = {FS6D: Few-Shot 6D Pose Estimation of Novel Objects},
journal = {CVPR},
year = {2022},
}